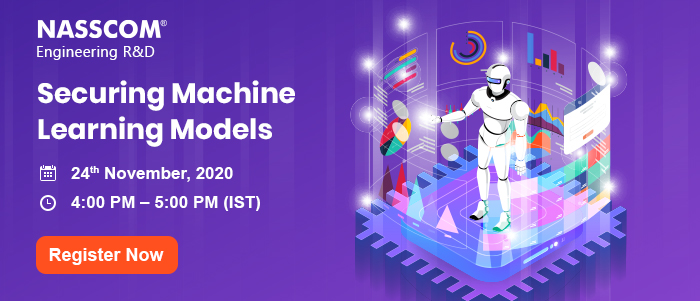 |
|
|
NASSCOM Engineering R&D is pleased to invite you for joining a webinar on "Securing Machine Learning Models" on 24th November, 2020 (Tuesday) from 04:00 PM - 05:00 PM (IST). |
|
ML models are popping up everywhere around us, be it e-commerce, networks or healthcare. We went through a journey of running these models on a local system to industrializing these models and scaling them to serve millions of users using cutting edge cloud technologies. However, very few people actually realize how easy or difficult these models are to hack & replicate using various black box & white box methodologies. |
|
This talk will walk you through important security aspects one has to keep in mind while deploying machine learning models on cloud, edge or on-premise. We will also showcase counter measures to defend these attacks as well. We share undertake the standard security expert's approach of a) Awareness b) Applicability c) Countermeasures. |
|
The talk will majorly focus attacks like: |
|
 |
Model extraction - How can an adversary replicate your model? |
|
 |
Model evasion / adversarial attacks - How can an adversary corrupt your model? |
|
 |
Model watermarking - How can one prove ownership of a model? |
|
|
|
The session would also showcase demo of these attacks on a variety of models and datasets, along with defense mechanisms. |
|
|
|
|
AGENDA |
|
|
Time
|
Speaker
|
Topic
|
5 mins
|
Overview & Context Setting |
NASSCOM ER&D Team |
45 mins
|
Session - Securing Machine Learning Models |
Raghotham Sripadraj - Senior Data Scientist, Ericsson GAIA
Rajib Biswas - Lead Data Scientist working, Ericsson GAIA
|
10 mins
|
Q&A Session
|
|
|
|
|
|
|
Speakers' Profile
|
|
|
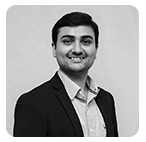 |
|
Raghotham Sripadraj Senior Data Scientist, Ericsson GAIA
|
|
|
|
|
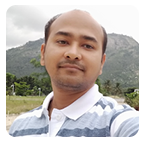 |
|
Rajib Biswas Lead Data Scientist working,
Ericsson GAIA
|
|
|
|
|
|
|
|
Registration |
|
Participation is free for all, but prior registration is mandatory. Click here to register.
|
|
|
|