Advisor & Researcher, AI Tech Ethics
In the dynamic AI landscape, responsible AI is pivotal for building trust, thus being the paramount for organizational success. This article emphasizes the need for trust-building and compliance with evolving RAI standards. This article outlines 10 actionable steps for organizations utilizing generative AI to initiate their responsible AI journey, promoting market alignment and ethical advancement.
In the burgeoning artificial intelligence (AI) landscape, the concept of responsible AI (RAI) emerges as a crucial value proposition. Organisations must prioritise the development of trust among stakeholders, recognising it as the cornerstone of their success. This involves actively aligning their practices with evolving RAI requirements within their customer segments or industries, tracking specific RAI trends, and establishing mechanisms for regulatory compliance, including European GDPR, US CCPA, EU AI Act, and Indian DPDP. By embracing responsible AI as an ongoing journey, organisations can fortify their market position and contribute to the responsible advancement of the AI technology ecosystem.
This article highlights the imperative of responsible AI for organisations developing or using generative AI technologies, while recommending 10 value-driven actions for them to launch their responsible AI journey:
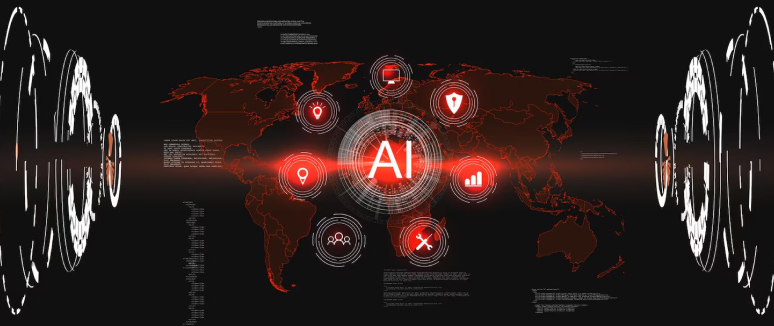
-
Understand the models in use better
For emerging generative AI-drive organisations, understanding and adopting key AI models like Large Language Model (LLM), Large Vision Model (LVM), Speech and Language Model (SLM), and Language and Audio Model (LAM) is essential. This entails not only understanding their capabilities but also acknowledging their inherent limitations. Organisations must understand dataset quality, training approaches, testing protocols, claimed performance, and associated failures of these models to prepare for robust implementation of their products. Anticipating downstream impacts from model deployment through proactive risk management and establishing testing protocols to gather potential shortcomings impacting customer solutions can support robust AI deployment. By pre-emptively addressing risks, organisations can uphold responsible AI practices, fostering trust and credibility among stakeholders while safeguarding against potential harm.
-
Establish and communicate policies
Establishing and communicating policies for responsible AI is one of the first steps to kick-start an organisation’s responsible AI journey. This entails crafting a responsible AI policy articulating the organisation's commitment to responsible AI practices aligned with fairness, transparency, and accountability. Care should be taken to consider industry standards and regulatory requirements in the environment where the organisations operate. Additionally, the organisations should define key aspects they aim to demonstrate in their responsible AI practices, including bias mitigation, data privacy assurance, transparency promotion, and inclusivity fostering. By setting clear expectations and guidelines, organisations can cultivate a culture of responsibility and integrity, empowering their teams with the knowledge and tools to uphold responsible AI principles effectively. These measures mitigate risks and build trust among stakeholders in the dynamic landscape of AI innovation.
-
Understand failure modes
To assess potential failure modes of the product, it is essential to understand the various contributing factors, including dependencies, data pipeline intricacies, context windows, and compute or service infrastructure. In addition, organisations should invest efforts in collating failures stemming from prompt engineering, model orchestration, optimisation (such as prompt compression or efficient fine-tuning), or domain-specific contexts. Furthermore, identifying potential security, safety, or fairness failures that might significantly impact the product's reliability or availability is crucial. To effectively manage these considerations, organisations must establish mechanisms to monitor and measure failures while instituting channels for users to report any encountered issues, thus fostering an opportunity to partner with the customers – to enhance transparency, accountability, and continuous product improvement for responsible AI.
-
Use practical tools for managing risks
Organisations may need to leverage various tools and techniques to effectively manage risks, including tools tailored to address specific challenges, while balancing the efforts based on latency, performance metrics, and cost implications. Furthermore, organisations must meticulously document any limitations or residual risks that may persist despite using these tools or techniques. By optimising tools, organisations can comprehensively identify, validate, monitor, and limit risks by employing multiple strategies, thus enhancing their ability to minimise potential adverse impacts.
-
Exhibit sensitivity towards diversity and inclusion
Organisations need to recognise that principles of diversity and inclusion extend beyond gender or race and should be contextualised to the specific use case environment served by the product. To align the product to the market's diversity or inclusion needs, the organisation must first identify distinct user groups that may demand or benefit from diverse and inclusive features or outcomes. It should accordingly plan for the diverse data, inclusive user interface, and experience design and ensure representation of diverse outcomes in such customer context. It is also necessary to validate any unaddressed user expectations regarding diversity and inclusion that may require additional efforts.
-
Engage with standards or industry bodies
Engaging with standards or industry bodies allows organisations to gain familiarity with emerging trends and contribute to nuanced insights around challenges or solutions in the market space. This includes actively participating in efforts to represent the complexity of risks in a domain or use case environment. By engaging in collaborative endeavours and contributing to industry forums, organisations gain valuable awareness and share experiences, thus playing a pivotal role in shaping responsible AI practices.
-
Enhance Terms of Use and EULA
Responsible AI practices are both internal and external to the organisation. Hence, organisations should explore ways to communicate and engage with customers regarding responsible AI efforts. This includes clearly articulating some of these efforts to protect and present them as part of their terms of use and End User License Agreements (EULA) for their product offerings. Organisations must diligently identify and clarify acceptable uses of their AI products, setting clear boundaries for users. Defining unacceptable uses and establishing mechanisms to track or report them is essential to upholding ethical standards and mitigate potential misuse. Moreover, setting performance thresholds ensures that AI systems operate within predetermined parameters, maintaining reliability and integrity. In addition, it is also essential to delineate clear responsibilities for different stakeholders involved in the supply chain to ensure accountability and transparency throughout the AI deployment process.
-
Use disclosure and disclaimer appropriately
While progressing in implementing responsible AI practices, it is crucial to use disclaimers and disclosures appropriately. Organisations should establish clear areas of disclosure to ensure that users and customers are well-informed about the product and its performance. This includes transparently communicating any limitations, risks, or potential biases associated with the technology. Additionally, it is essential to enumerate areas where users and customers are expected to leverage the tool at their own risk or where the performance may not be optimal. By providing clear and comprehensive disclaimers and disclosures, organisations can promote transparency, empower users to make informed decisions and mitigate the potential for misunderstandings or dissatisfaction.
-
Diversify responsibility
Diversifying responsibility for risks arising from the tool should be among the key strategies product organisations should consider. This involves strategically incorporating a "Human in the Loop" approach or human intervention within products, especially when automation may prove inefficient or unreliable or where human input offers a superior value than automation alone. By recognising the nuanced strengths and limitations of automated systems and human decision-making, organisations can foster a synergistic collaboration that maximises efficiency and reliability, ultimately, the quality of outcomes. This approach enhances the robustness and adaptability of AI solutions and supports trustworthy alignment with user needs and expectations.
-
Demonstrate and explore opportunities to create value from RAI efforts
Trade efforts around responsible AI encompass presenting RAI initiatives as a compelling value proposition while leveraging them to differentiate products in the market and mitigate risks. By positioning RAI efforts as a value proposition, organisations demonstrate their commitment to ethical and responsible practices, enhancing their brand reputation, and fostering stakeholder trust. Furthermore, highlighting RAI product features serves as a means for market differentiation, setting offerings apart from competitors and appealing to conscientious consumers. Not the least, integrating RAI functionalities can also act as a risk reduction solution, mitigating potential ethical, legal, and reputational risks associated with AI technologies.
Through these strategic efforts, organisations can bolster their market presence and contribute to the advancement of responsible AI practices in the industry. By evolving with progressive steps towards responsible AI, organisations can enhance their value proposition while contributing to the ethical and sustainable advancement of AI technology.
Keywords: Responsible AI, RAI, AI ethics, Customer centricity, AI, artificial Intelligence, Generative AI, GenAI, Technology, Innovation, Large Language Models (LLMs), Large Action Models (LAMs), Opinion